- Chapter 1 : Introduction
- 1.1. Normative Motivation
- 1.2. Empirical Motivations
- 1.2.1. Monetary Vs. Non-Monetary Household Deprivations
- 1.2.2. Trends in Monetary Poverty Vs. Trends in Non-Monetary Deprivations
- 1.2.3. Associations across Non-Monetary Deprivations
- 1.2.4. Economic Growth and Social Indicators
- 1.2.5. The Value-addded of Joint Distribution of Deprivations: Clustering and Identification
- 1.2.6. Data and Computational Technologies
- 1.3. Policy Motivation
- 1.4. Content and Structure
- 1.5. How to Use this Book
- Chapter 2 : The Framework
- 2.1. Review of Unidimensional Measurement and FGT Measures
- 2.1.1. Identification of the Income Poor
- 2.1.2. Aggregation of the Income Poor
- 2.2. Notation and Preliminaries for Multidimensional Poverty Measurement
- 2.2.1. Identifying Deprivations
- 2.2.2. Identification and Aggregation in the Multidimensional Case
- 2.2.3. The Joint Distribution
- 2.2.4. Marginal Methods
- 2.2.5. Useful Matrix and Vector Operations
- 2.3. Scales of Measurement: Ordinal and Cardinal Data
- 2.4. Comparability across People and Dimensions
- 2.5. Properties for Multidimensional Poverty Measures
- 2.5.1. Invariance Properties
- 2.5.2. Dominance Properties
- 2.5.3. Subgroup Properties
- 2.5.4. Technical Properties
- 2.1. Review of Unidimensional Measurement and FGT Measures
- Chapter 3 : Overview of Methods for Multidimensional Poverty Assessment
- 3.1. Dashboard of Indicators and Composite Indices
- 3.2. Venn Diagrams
- 3.2.1. The Methodology and Applications
- 3.2.2. A Critical Evaluation
- 3.3. The Dominance Approach
- 3.3.1. Poverty Dominance in Unidimensional Framework
- 3.3.2. Poverty Dominance in the Multidimensional Framework
- 3.3.3. Applications of the Multidimensional Dominance Approach
- 3.3.4. A Critical Evaluation
- 3.4. Statistical Approaches
- 3.4.1. Sub-Steps in Aggregation within Multivariate Statistical Methods
- 3.4.2. Applications of Statistical Approaches in Poverty Aggregation
- 3.4.3. A Brief and Formal Outline of Different Statistical Approaches
- 3.4.4. A Critical Evaluation
- 3.5. Fuzzy Set Approaches
- 3.5.1. Fuzzy Set Poverty Approach
- 3.5.2. Membership Functions
- 3.5.3. Aggregation across Dimensions
- 3.5.4. Aggregation across People
- 3.5.5. A Critical Evaluation
- 3.6. Axiomatic Measures
- 3.6.1. Measures Based on a Counting Approach
- 3.6.2. Measures Using an Aggregate Achievement Approach
- 3.6.3. A Critical Evaluation
- Chapter 4 : Counting Approaches: Definitions, Origins and Implementations
- 4.1. Definition and Origins
- 4.2. Measures of Deprivation in Europe and their Influence
- 4.3. Measures of Unsatisfied Basic Needs in Latin America & Beyond
- 4.3.1. Counting Approaches in Measures of Child Poverty
- 4.4. Counting Approaches in Targeting
- 4.5. Final Comments on Counting Approaches
- Chapter 5 : The Alkire-Foster Counting Methodology
- 5.1. The AF Class of Poverty Measures: Overview and Practicality
- 5.2. Identification of the Poor: The Dual-Cutoff Approach
- 5.2.1. The Deprivation Cutoffs: Identifying Deprivations and Obtaining Deprivation Scores
- 5.2.2. Alternative Notation and Presentation
- 5.2.3. The Second Cutoff: Identifying the Poor
- 5.2.4. Dual-cutoff Approach and Censoring
- 5.3. Aggregation: The Adjusted Headcount Ratio
- 5.4. Distinctive Characteristics of the Adjusted Headcount Ratio
- 5.5. The set of Consistent Partial Indices of the Adjusted Headcount Ratio
- 5.5.1. Incidence and Intensity of Poverty
- 5.5.2. Subgroup Decomposition
- 5.5.3. Dimensional Breakdown
- 5.6. A Case Study: The Global Multidimensional Poverty Index (MPI)
- 5.7. AF Class Measures Used with Cardinal Variables
- 5.7.1. The Normalized Gap Matrix
- 5.7.2. The Adjusted Poverty Gap, Adjusted FGT, and M Measures
- 5.8. Some implementations of the AF Methodology
- Chapter 6 : Normative Choices in Measurement Design
- 6.1. The Adjusted Headcount Ratio: A Measure of Capability Poverty?
- 6.2. Kinds of Normative Choices
- 6.3. Elements of Measurement Design
- 6.3.1. Purpose(s) of a Measure
- 6.3.2. Choice of Space
- 6.3.3. Units of Identification and Analysis
- 6.3.4. Dimensions
- 6.3.5. Indicators
- 6.3.6. Deprivation Cutoffs
- 6.3.7. Weights
- 6.3.8. Poverty Cutoff k
- 6.4. Concluding Reflections
- Chapter 7 : Data and Analysis
- 7.1. Data for Multidimensional Poverty Measurement
- 7.2. Issues in Indicator Design
- 7.2.1. Unit-level Indicator Accuracy
- 7.2.2. Indicator Transformation to Match Unit of Identification
- 7.2.3. The Unit of Identification and Applicable Population
- 7.2.4. Assessing Combined Measures
- 7.2.5. Handling Missing Values
- 7.3. Relationships among Indicators
- Chapter 8 : Robustness Analysis and Statistical Inference
- 8.1. Robustness Analysis
- 8.1.1. Dominance Analysis for Changes in the Poverty Cutoff
- 8.1.2. Rank Robustness Analysis
- 8.2. Statistical Inference
- 8.2.1. Standard Errors
- 8.2.2. Confidence Intervals
- 8.2.3. Hypothesis Tests
- 8.3. Robustness Analysis with Statistical Inference
- 8.3.1. Appendix: Methods for Computing Standard Errors
- 8.1. Robustness Analysis
- Chapter 9 : Distribution and Dynamics
- 9.1. Inequality among the Poor
- 9.1.1. Integrating Inequality into Poverty Measures
- 9.1.2. Analyzing Inequality Separately: A Descriptive Tool
- 9.1.3. Using a Separate Inequality Measure
- 9.2. Descriptive Analysis of Changes over Time
- 9.2.1. Changes in M0, H and A across Two Time Periods
- 9.2.2. An Example: Analyzing Changes in Global MPI for Four Countries
- 9.2.3. Population Growth and Change in the Number of Multidimensionally Poor
- 9.2.4. Dimensional Changes (Uncensored and Censored Headcount Ratios)
- 9.2.5. Subgroup Decomposition of Change in Poverty
- 9.3. Changes Over Time by Dynamic Subgroups
- 9.3.1. Exits, Entries, and the Ongoing Poor: A Two Period Panel
- 9.3.2. Decomposition by Incidence and Intensity for Cross-Sectional Data
- 9.3.3. Theoretical Incidence-Intensity Decompositions
- 9.4. This Complementary Chronic Poverty with Multiple Time Periods
- 9.4.1. Chronic Poverty Measurement Using Panel Data
- 9.4.2. Properties
- 9.4.3. Consistent Partial Indices
- 9.4.4. Poverty Transitions Using Panel Data
- 9.1. Inequality among the Poor
- Chapter 10 Some Regression models for AF measures
- 10.1. Micro and Macro Regressions
- 10.2. Generalized Linear Models
- 10.2.1. Classic Linear Regression
- 10.2.2. The Generalization
- 10.2.3. Estimation and Goodness of Fit
- 10.3. Micro Regression Models with AF Measures
- 10.3.1. A micro-regression example
- 10.4. Macro Regression Models for M0 and H
- 10.4.1. Modelling M0 or H
- 10.4.2. Econometric issues for an empirical model of M0 or H
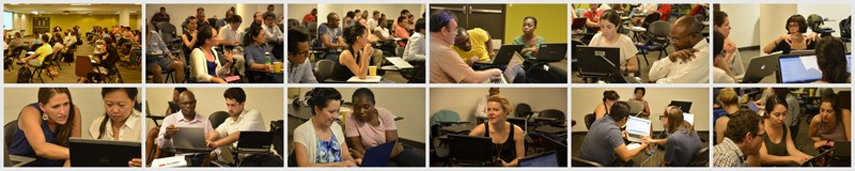